Good, but not always Fair: An Evaluation of Gender Bias for three Commercial Machine Translation Systems
DOI:
https://doi.org/10.7146/hjlcb.vi63.137553Keywords:
Machine Translation, Gender bias, evaluationAbstract
Machine Translation (MT) continues to make significant strides in quality and is increasingly adopted on a larger scale. Consequently, analyses have been redirected to more nuanced aspects, intricate phenomena, as well as potential risks that may arise from the widespread use of MT tools. Along this line, this paper offers a meticulous assessment of three commercial MT systems - Google Translate, DeepL, and Modern MT - with a specific focus on gender translation and bias. For three language pairs (English-Spanish, English-Italian, and English-French), we scrutinize the behavior of such systems at several levels of granularity and on a variety of naturally occurring gender phenomena in translation. Our study takes stock of the current state of online MT tools, by revealing significant discrepancies in the gender translation of the three systems, with each system displaying varying degrees of bias despite their overall translation quality.
References
Alhafni B., Obeid O., & Habash N. (2023). The User-Aware Arabic Gender Rewriter. Proceedings of the First Workshop on Gender-Inclusive Translation Technologies, 3–11, Tampere, Finland. European Association for Machine Translation.
Bender E. M. & Friedman B. (2018). Data Statements for Natural Language Processing: Toward Mitigating System Bias and Enabling Better Science. Transactions of the Association for Computational Linguistics, 6, 587–604.
Bentivogli L, Bisazza A., Cettolo M., & Federico M. (2016). Neural versus Phrase-Based Machine Translation Quality: a Case Study. Proceedings of the 2016 Conference on Empirical Methods in Natural Language Processing, 257–267, Austin, Texas. Association for Computational Linguistics.
Bentivogli L., Savoldi B., Negri M., Di Gangi M. A., Cattoni R., & Turchi M. (2020). Gender in Danger? Evaluating Speech Translation Technology on the MuST-SHE Corpus. Proceedings of the 58th Annual Meeting of the Association for Computational Linguistics, 6923–6933, Online. Association for Computational Linguistics. https://aclanthology.org/2020.acl-main.619
Callison-Burch C., Osborne M., & Koehn P. (2006). Re-evaluating the Role of Bleu in Machine Translation Research. 11th Conference of the European Chapter of the Association for Computational Linguistics, 249–256, Trento, Italy. Association for Computational Linguistics.
Chorowski, J. K., Bahdanau, D., Serdyuk, D., Cho, K., & Bengio, Y. (2015). Attention-based models for speech recognition. Advances in neural information processing systems, 28(1), 577-585.
Choubey P. K., Currey A., Mathur P., & Dinu G. (2021). GFST: Gender-Filtered Self-Training for More Accurate Gender in Translation. Proceedings of the 2021 Conference on Empirical Methods in Natural Language Processing, 1640–1654, Online and Punta Cana, Dominican Republic. Association for Computational Linguistics.
Corbett, G. G. (2013). Gender typology. The expression of gender, 87-130.
Crawford K. [Steven Van Vaerenbergh]. (2017, December 18). The Trouble with Bias (NIPS 2017 keynote) [Video]. YouTube. https://www.youtube.com/watch?v=ggzWIipKraM.
Currey A., Nadejde M., Pappagari R. R., Mayer M., Lauly S., Niu X., Hsu B., & Dinu G. (2022). MT-GenEval: A Counterfactual and Contextual Dataset for Evaluating Gender Accuracy in Machine Translation. Proceedings of the 2022 Conference on Empirical Methods in Natural Language Processing, 4287–4299, Abu Dhabi, United Arab Emirates. Association for Computational Linguistics.
Curzan A. (2003). Gender Shifts in the History of English. Cambridge University Press.
Escolano C., Ojeda G., Basta C., & Costa-jussa M. R. (2021). Multi-Task Learning for Improving Gender Accuracy in Neural Machine Translation. Proceedings of the 18th International Conference on Natural Language Processing (ICON), 12–17, National Institute of Technology Silchar, Silchar, India. NLP Association of India (NLPAI). https://aclanthology.org/2021.icon-main.3.
Gaido M., Savoldi B., Bentivogli L., Negri M., & Turchi M. (2020). Breeding Gender-aware Direct Speech Translation Systems. Proceedings of the 28th International Conference on Computational Linguistics, 3951–3964, Barcelona, Spain (Online). International Committee on Computational Linguistics. https://aclanthology.org/2020.coling-main.350.
Germann U., Barbu E., Bentivogli L., Bertoldi N., Bogoychev N., Buck C., Caroselli D., Carvalho L., Cattelan A., Cettolo R., Federico M., Haddow B., Madl D., Mastrostefano L., Mathur P., Ruopp A., Samiotou A., Sudharshan V., Trombetti M., et al. (2016). ModernMT: a new open-source machine translation platform for the translation industry. Proceedings of the 19th Annual Conference of the European Association for Machine Translation: Projects/Products, Riga, Latvia. Baltic Journal of Modern Computing.
Gygax P. M., Gabriel U., Sarrasin O., Oakhill J., & Garnham A. (2008). Generically intended, but specifically interpreted: When beauticians, musicians and mechanics are all men. Language and Cognitive Processes, 23, 464–485.
Hamilton M. C. (1988). Using masculine generics: Does generic he increase male bias in the user’s imagery? Sex roles, 19 (11–12), 785–799.
Hovy D. & Spruit S. L. (2016). The Social Impact of Natural Language Processing. Proceedings of the 54th Annual Meeting of the Association for Computational Linguistics (Volume 2: Short Papers), 591–598, Berlin, Germany. Association for Computational Linguistics.
Johnson M., Schuster M., Le Q. V., Krikun M., Wu Y., Chen Z., Thorat N., Viégas F., Wattenberg M., Corrado G., Hughes M., & Dean J. (2017). Google’s Multilingual Neural Machine Translation System: Enabling Zero-Shot Translation. Transactions of the Association for Computational Linguistics, 5, 339–351.
Kreiner H., Sturt P., & Garrod S. (2008). Processing definitional and stereotypical gender in reference resolution: Evidence from eye-movements. Journal of Memory and Language, 58, 239–261.
Kocmi T., Federmann C., Grundkiewicz R., Junczys-Dowmunt M., Matsushita H., & Menezes A. (2021). To Ship or Not to Ship: An Extensive Evaluation of Automatic Metrics for Machine Translation. Proceedings of the Sixth Conference on Machine Translation, 478–494, Online. Association for Computational Linguistics.
Konishi, T. (1993). The semantics of grammatical gender: A cross-cultural study. Journal of psycholinguistic research, 22, 519-534.
Kuczmarski, J., & Johnson, M. (2018, December 6). Gender-aware natural language translation. Google Blog [online]. https://www.blog.google/products/translate/reducing-gender-bias-google-translate/.
Levy S., Lazar K., & Stanovsky G. (2021). Collecting a Large-Scale Gender Bias Dataset for Coreference Resolution and Machine Translation. Findings of the Association for Computational Linguistics: EMNLP 2021, 2470–2480, Punta Cana, Dominican Republic. Association for Computational Linguistics.
Lardelli, M., & Gromann, D. (2022). Gender-Fair (Machine) Translation. Proceedings of the New Trend in Translation & Technology Conference, 166-177.
Monti, J. (2017). Questioni di genere in traduzione automatica. Al femminile. Scritti linguistici in onore di Cristina Vallini, 139, 411-431.
Monti J. (2020). Gender issues in machine translation: An unsolved problem? The Routledge Handbook of Translation, Feminism and Gender, 457-468. Routledge.
Naik R., Rarrick S., Poudel S., Mathur V., Chandrala J. K., Mohan C., Schwartz L., Nguyen S., Bhagwat A., & Chowdhary V. (2023, March 8). Bing's gendered translations tackle bias in translation. Microsoft Translator Blog [online]. https://www.microsoft.com/en-us/translator/blog/2023/03/08/bings-gendered-translations-tackle-bias-in-translation/.
Nissen, U. K. (2002). Aspects of translating gender. Linguistik online, 11(2), 25-37.
Nissim M., Pannitto L. (2022). Che cos’è la linguistica computazionale. Carocci, Roma.
Och, F. (2006, April 28). Statistical machine translation live – Google AI Blog. Google AI Blog [online]. https://ai.googleblog.com/2006/04/statistical-machine-translation-live.html.
Papineni, K., Roukos, S., Ward, T., & Zhu, W. (2002). BLEU: A Method for Automatic Evaluation of Machine Translation. Proceedings of the 40th Annual Meeting of the Association for Computational Linguistics, 311-318. Association for Computational Linguistics.
Pichai S. [Google for Developers]. (2016, May 18). Google I/O 2016 ー Keynote [Video]. YouTube. https://www.youtube.com/watch?v=862r3XS2YB0.
Pitman, J. (2021, April 28). Google Translate: One billion installs, one billion stories. Google Blog. https://blog.google/products/translate/one-billion-installs/.
Post M. (2018). A Call for Clarity in Reporting BLEU Scores. Proceedings of the Third Conference on Machine Translation: Research Papers, 186–191, Brussels, Belgium. Association for Computational Linguistics.
Prates, M. O., Avelar, P. H., & Lamb, L. C. (2020). Assessing gender bias in machine translation: a case study with Google translate. Neural Computing and Applications, 32, 6363-6381.
Rarrick, S., Naik, R., Mathur, V., Poudel, S., & Chowdhary, V. (2023). GATE: A Challenge Set for Gender-Ambiguous Translation Examples.
Rescigno A. A., Monti J., Way A., & Vanmassenhove E. (2020). A Case Study of Natural Gender Phenomena in Translation: A Comparison of Google Translate, Bing Microsoft Translator and DeepL for English to Italian, French and Spanish. Workshop on the Impact of Machine Translation (iMpacT 2020), 62–90, Virtual. Association for Machine Translation in the Americas.
Renduchintala A. & Williams A. (2022). Investigating Failures of Automatic Translation in the Case of Unambiguous Gender. Proceedings of the 60th Annual Meeting of the Association for Computational Linguistics (Volume 1: Long Papers), 3454–3469, Dublin, Ireland. Association for Computational Linguistics.
Saunders D. & Byrne B. (2020). Reducing Gender Bias in Neural Machine Translation as a Domain Adaptation Problem. Proceedings of the 58th Annual Meeting of the Association for Computational Linguistics, 7724–7736, Online. Association for Computational Linguistics.
Sánchez, E., Andrews, P., Stenetorp, P., Artetxe, M., & Costa-jussà, M. R. (2023). Gender-specific Machine Translation with Large Language Models. arXiv preprint arXiv:2309.03175.
Savoldi B., Gaido M., Bentivogli L., Negri M., & Turchi M. (2021). Gender Bias in Machine Translation. Transactions of the Association for Computational Linguistics, 9, 845–874.
Savoldi, B., Gaido, M., Bentivogli, L., Negri, M., & Turchi, M. (2022). Under the Morphosyntactic Lens: A Multifaceted Evaluation of Gender Bias in Speech Translation. Proceedings of the 60th Annual Meeting of the Association for Computational Linguistics (Volume 1: Long Papers), 1807-1824. Association for Computational Linguistics.
Schiebinger L. (2014). Scientific research must take gender into account. Nature, 507 (7490), 9-9.
Sharma S., Dey M., & Sinha K. (2022). How sensitive are translation systems to extra contexts? Mitigating gender bias in Neural Machine Translation models through relevant contexts. Findings of the Association for Computational Linguistics: EMNLP 2022, 1968–1984, Abu Dhabi, United Arab Emirates. Association for Computational Linguistics.
Stafanovičs A., Bergmanis T., & Pinnis M. (2020). Mitigating Gender Bias in Machine Translation with Target Gender Annotations. Proceedings of the Fifth Conference on Machine Translation, 629–638, Online. Association for Computational Linguistics.
Stahlberg, D., Braun, F., Irmen, L., & Sczesny, S. (2007). Representation of the sexes in language. In K. Fiedler (Ed.), Social communication, 163–187. Psychology.
Stanovsky G., Smith N. A., & Luke Zettlemoyer. (2019). Evaluating Gender Bias in Machine Translation. Proceedings of the 57th Annual Meeting of the Association for Computational Linguistics, 1679–1684, Florence, Italy. Association for Computational Linguistics.
Sun T, Gaut A., Tang S., Huang Y., ElSherief M., Zhao J., Mirza D., Belding E., Chang K-W., & Yang Wang W. (2019). Mitigating Gender Bias in Natural Language Processing: Literature Review. Proceedings of the 57th Annual Meeting of the Association for Computational Linguistics, 1630–1640, Florence, Italy. Association for Computational Linguistics.
Sutskever, I., Vinyals, O., & Le, Q. V. (2014). Sequence to sequence learning with neural networks. Advances in neural information processing systems, 27, 3104-3112.
Tavosanis, S. P. M. (2020). Valutazione umana di DeepL a livello di frase per le traduzioni di testi specialistici dall’inglese verso l’italiano. Computational Linguistics CLiC-it 2020, 422.
Toral A. & Sánchez-Cartagena V. M. (2017). A Multifaceted Evaluation of Neural versus Phrase-Based Machine Translation for 9 Language Directions. Proceedings of the 15th Conference of the European Chapter of the Association for Computational Linguistics: Volume 1, Long Papers, 1063–1073, Valencia, Spain. Association for Computational Linguistics.
Ullmann, S. (2022). Gender Bias in Machine Translation Systems. In Hanemaayer, A. (eds.) Artificial Intelligence and Its Discontents. Social and Cultural Studies of Robots and AI. Palgrave Macmillan, Cham.
Vanmassenhove E., Hardmeier C., & Way A. (2018). Getting Gender Right in Neural Machine Translation. Proceedings of the 2018 Conference on Empirical Methods in Natural Language Processing (EMNLP), 3003–3008, Brussels, Belgium, November–October.
Vashee, K. (2021a, September 27). A Closer Look at ModernMT. ModernMT Blog [online]. https://blog.modernmt.com/modernmt-a-closer-look-at-an-emerging-enterprise-mt-powerhouse/.
Vashee, K. (2021b, October 25). Understanding MT Quality: BLEU Scores. ModernMT Blog [online]. https://blog.modernmt.com/understanding-mt-quality-bleu-scores.
Vaswani, A., Shazeer, N., Parmar, N., Uszkoreit, J., Jones, L., Gomez, A. N., et al. (2017). Attention is all you need. 31st Conference on Neural Information Processing Systems (NIPS 2017), 5998-6008, Long Beach, CA, USA.
Vieira, N. L, O’Sullivan, C., Zhang, X., & O’Hagan, M. (2022) Machine translation in society: insights from UK users. Language Resources and Evaluation, 1-22.
Wu, Y., Schuster, M., Chen, Z., Le, Q. V., Norouzi, M., Macherey, W., Krikun, M., Cao, Y., Gao, Q., Macherey, K. et al. (2016). Google's neural machine translation system: Bridging the gap between human and machine translation. Computing Research Repository.
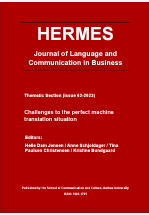
Downloads
Published
How to Cite
Issue
Section
License
Copyright (c) 2024 Silvia Alma Piazzolla, Beatrice Savoldi, Luisa Bentivogli

This work is licensed under a Creative Commons Attribution 4.0 International License.
Authors who publish with this journal agree to the following terms:
a. Authors retain copyright and grant the journal right of first publication with the work simultaneously licensed under a Creative Commons Attribution License that allows others to share the work with an acknowledgement of the work's authorship and initial publication in this journal.
b. Authors are able to enter into separate, additional contractual arrangements for the non-exclusive distribution of the journal's published version of the work (e.g., post it to an institutional repository or publish it in a book), with an acknowledgement of its initial publication in this journal.
c. Authors are permitted and encouraged to post their work online (e.g., in institutional repositories or on their website) prior to and during the submission process, as it can lead to productive exchanges, as well as earlier and greater citation of published work (See The Effect of Open Access).